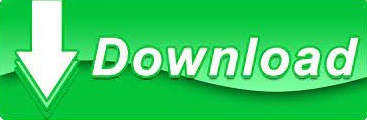

But many nonlinear problems are non-convex and are likely to have multiple locally optimal solutions, as in the chart below.
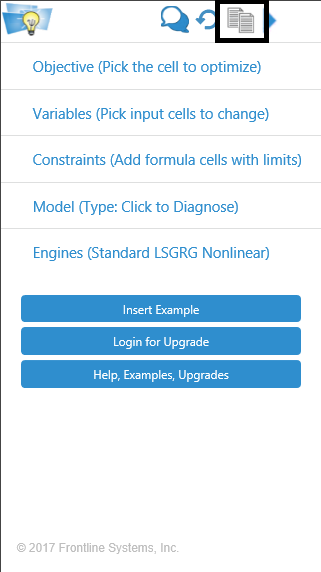
These include LP problems QP problems where the objective is positive definite (if minimizing negative definite if maximizing) and NLP problems where the objective is a convex function (if minimizing concave if maximizing) and the constraints form a convex set. In convex optimization problems, a locally optimal solution is also globally optimal. You can picture this as a point at the top of a "peak" or at the bottom of a "valley" which may be formed by the objective function and/or the constraints - but there may be a higher peak or a deeper valley far away from the current point. A locally optimal solution is one where there are no other feasible solutions "in the vicinity" with better objective function values. A globally optimal solution is one where there are no other feasible solutions with better objective function values.
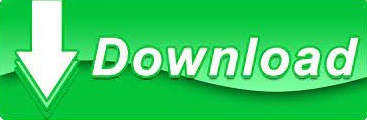